Combining your process data with hardwired knowledge
AI and machine learning (ML) are powerful, disruptive technologies. However, they require high volumes of data to be reliable — a process development challenge where datasets are scarce.
DataHow solves this by creating hybrid models that fuse a hard-coded “mechanistic” backbone, which contains known process dynamics, with machine learning models that continually learn from new process data.
These structured yet flexible models provide process scientists with unparalleled, reliable insight and prediction power. DataHow also dynamically uses hybrid models to support iterative experimental design and planning.
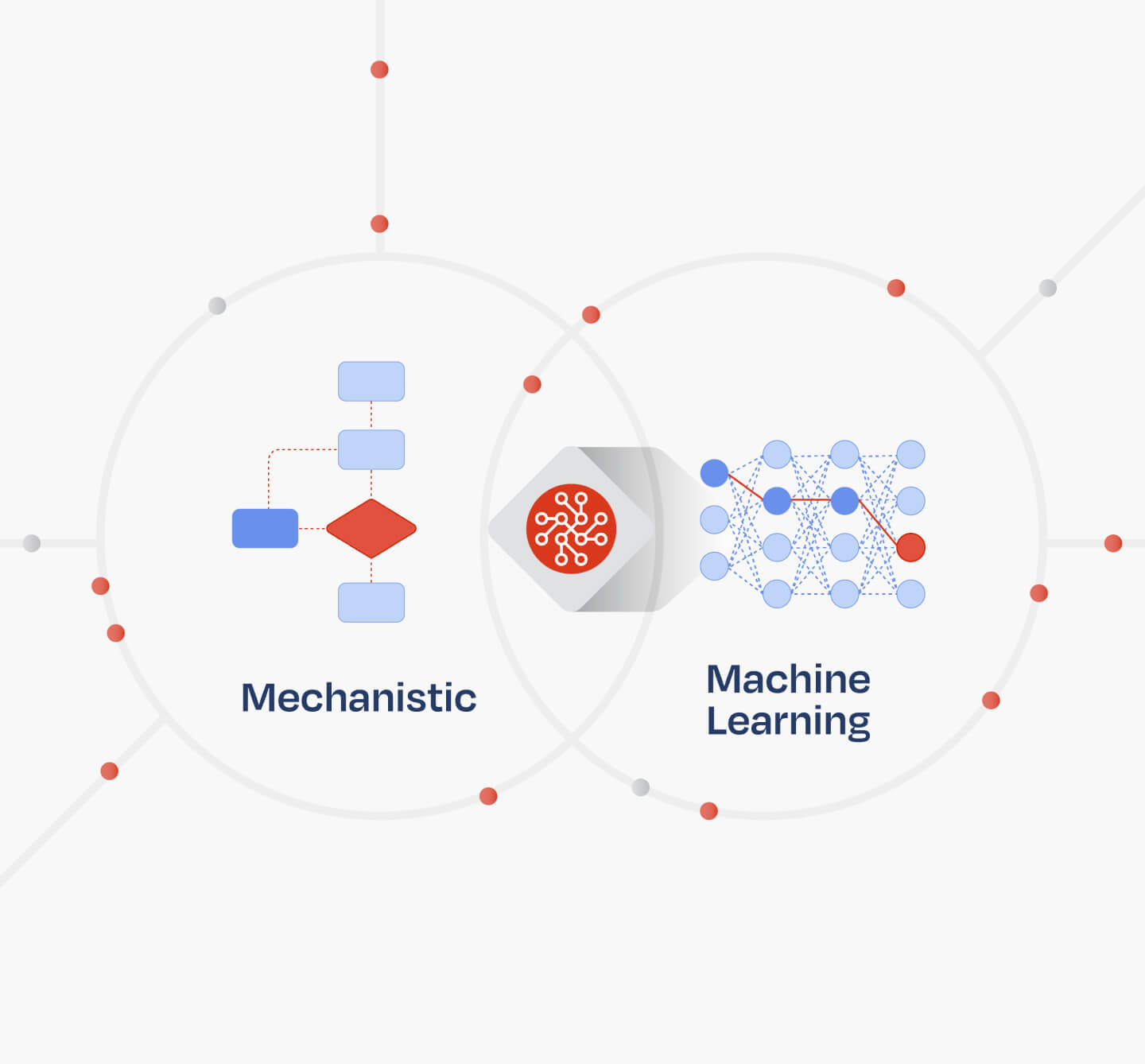
Key Benefits
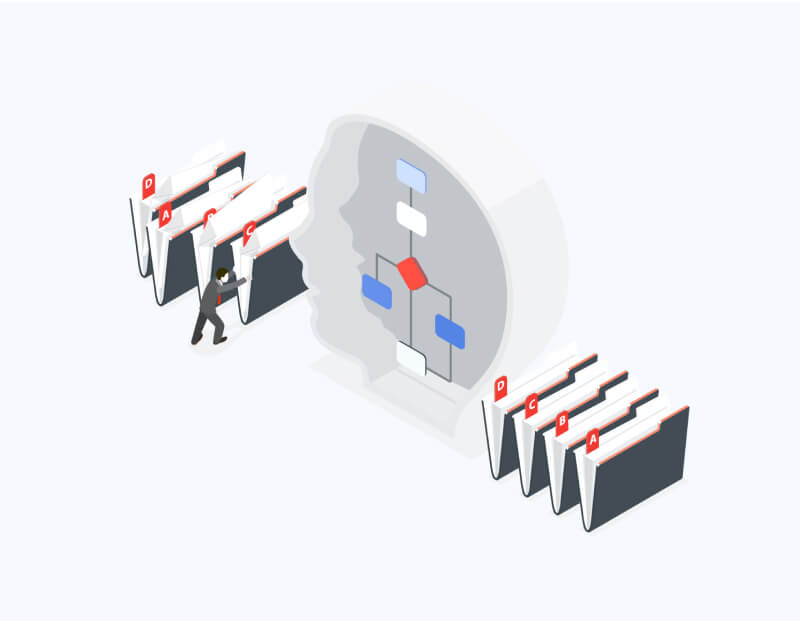
Hybrid models use ML and pre-programmed bioprocess knowledge to understand complex process dynamics and patterns
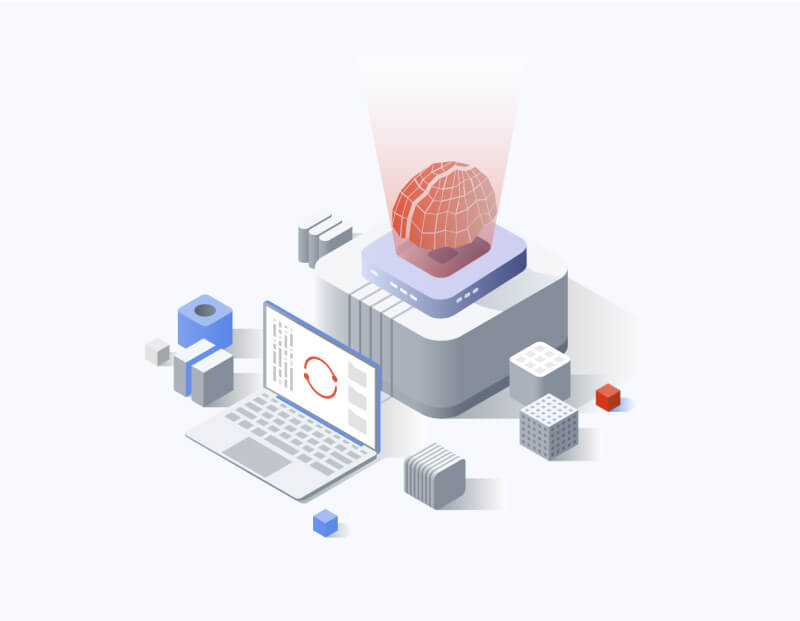
Uses hybrid models to learn and support critical process development tasks and decisions iteratively
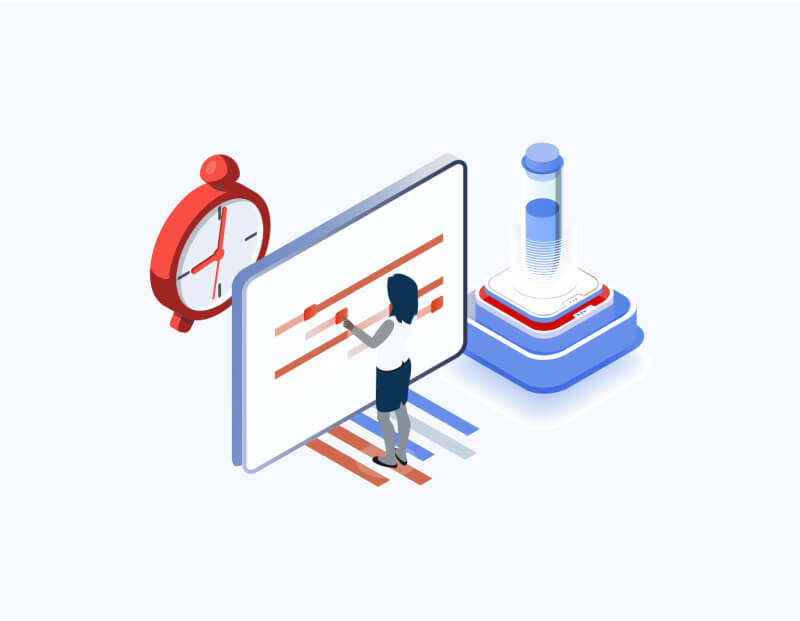
Maximizes insights from each experiment and reduces experimental requirements