DataHow’s
AI Approach
A new era for bioprocess data analytics and development
Bioprocess data analytics hasn’t significantly changed since the introduction of Design of Experiment (DoE) in the 1960s. The application of AI technologies is changing this. Purely statistical models remain inadequate to understand the complexities of bioprocesses; hybrid AI models that combine hardwired and AI technology are able to provide bioprocess scientists with the tools to generate unparalleled process insights.
DoE, a revolution in its time, helped scientists by providing a structured approach to process development – but it has its limitations when faced with the complex non-linear dynamics within bioprocesses. When applied to complex processes (high-dimensional design spaces), the number of experiments required becomes impractical, costly, and time-consuming.
DataHow, by harnessing AI technologies, proposes an innovative approach to DoE and process optimization.
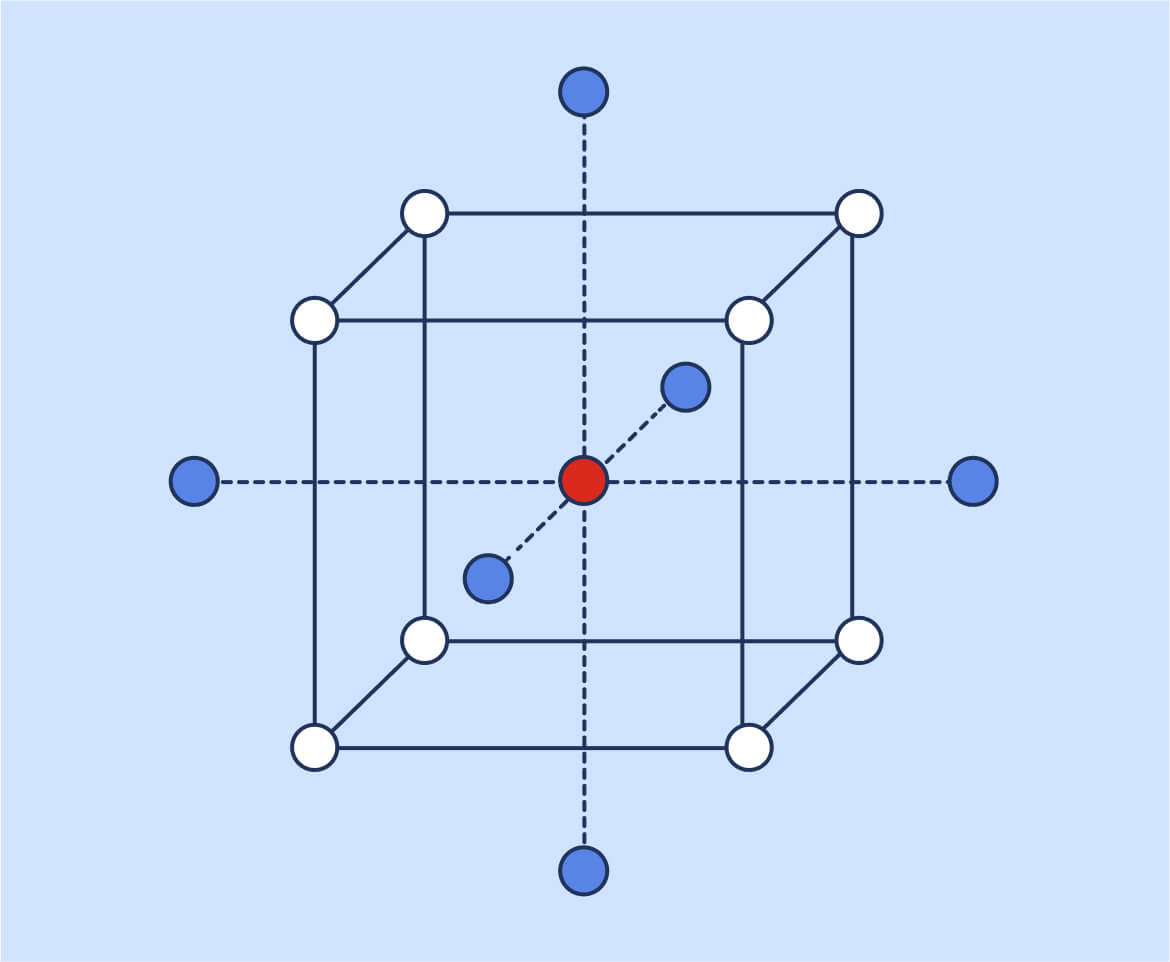
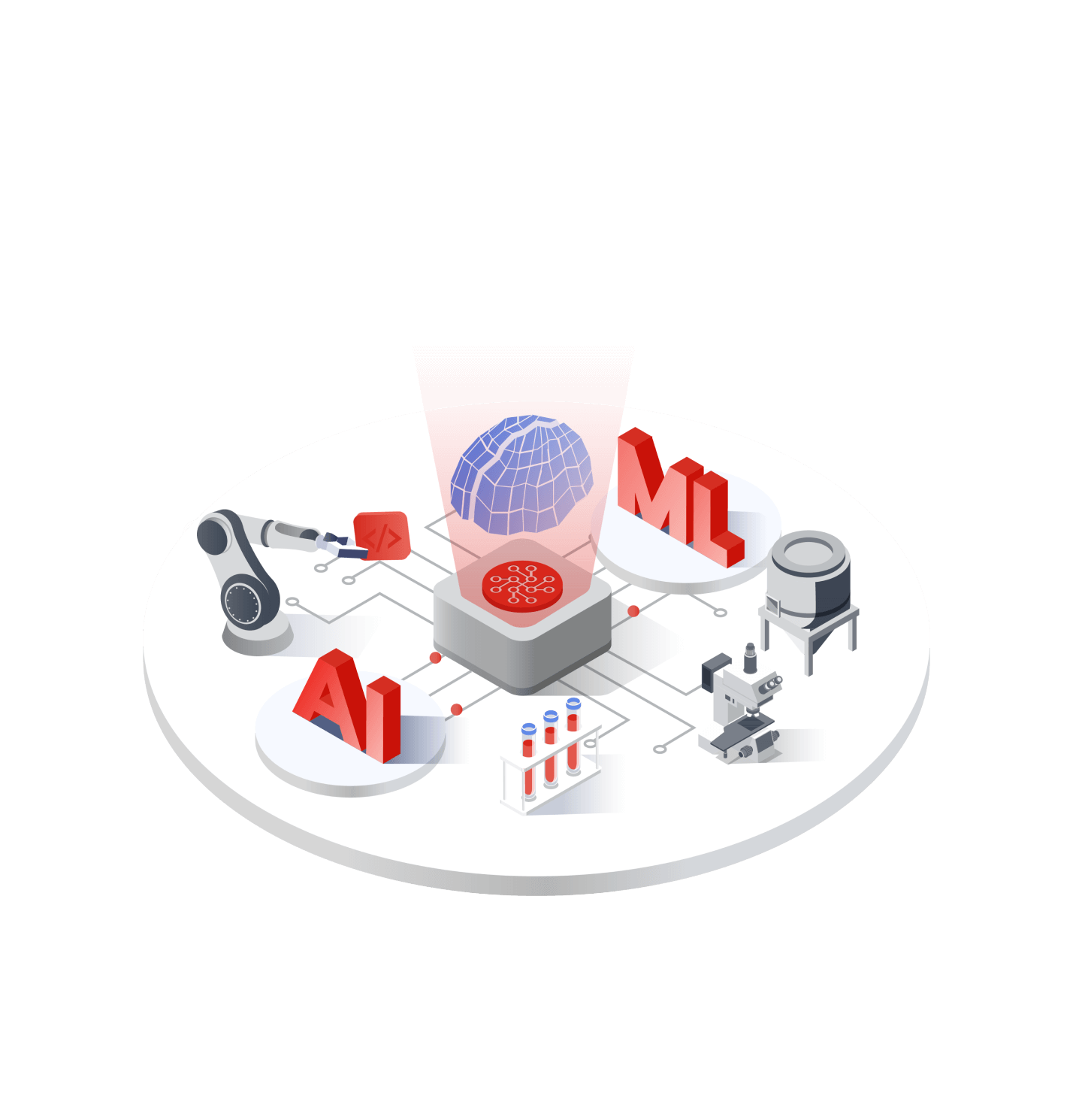
Hybrid models enabling faster learning of bioprocesses
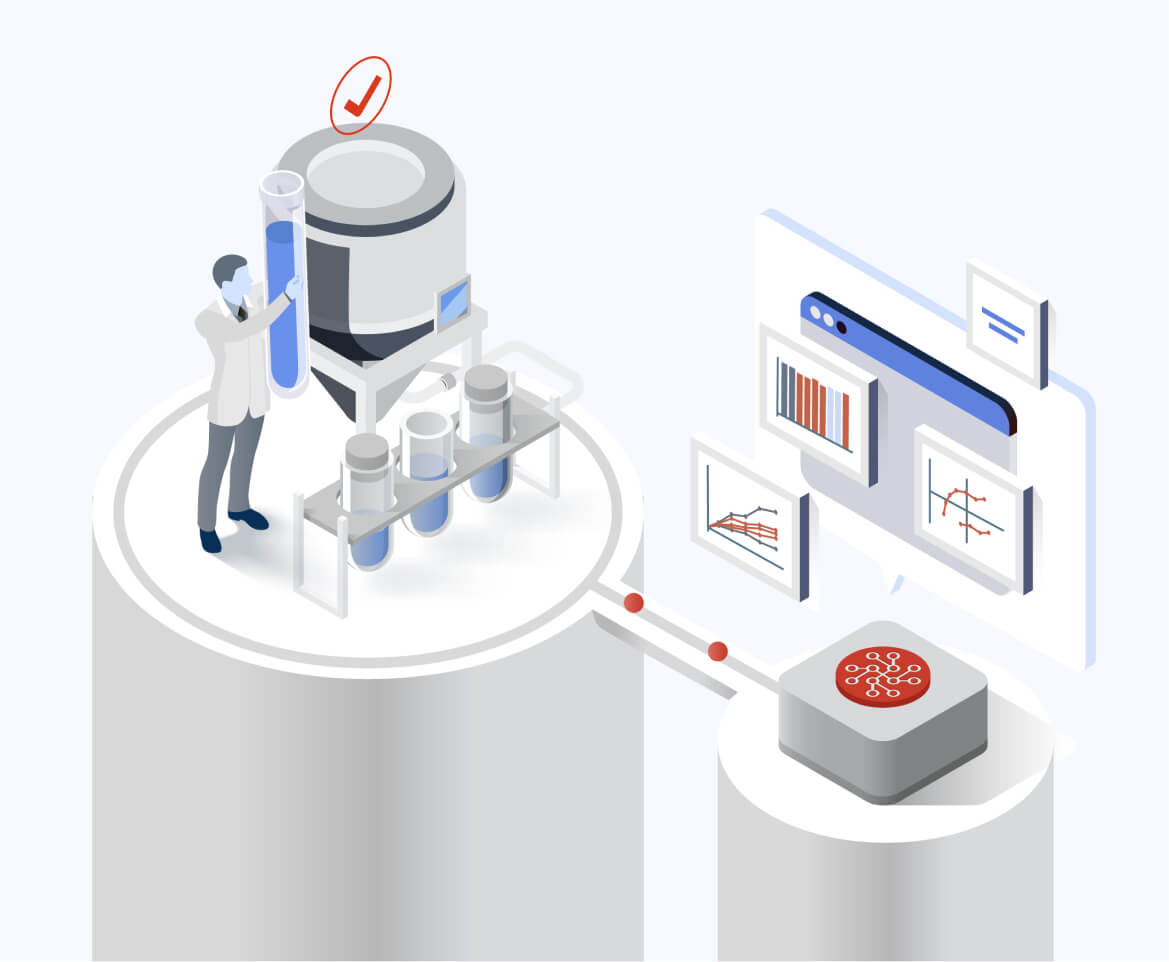
Iterative experimental design and targeted, efficient exploration
These technologies and methods are embedded within DataHowLab, allowing process development teams to independently benefit from this approach and take one step closer to pharma 4.0.
Meet with one of our bioprocess development experts for a demo of DataHowLab and see how holistic digital modeling, data analysis, and data management can transform your operation.