Stronger CQA prediction with fewer experiments with DataHowLabs Hybrid Models
DataHow collaborated with Bristol Mayers Squibb (BMS) to evaluate experimental data from a complex upstream mAbs bioprocess and the ability of DataHow’s AI-enabled, hybrid process models to accurately predict critical quality attributes (CQAs) compared to industry state-of the-art “black box” models.
The impact was clear – the hybrid models delivered greater process insight, leading to substantially stronger CQA prediction, while requiring less experimental data.
–
This case study is further detailed in a published work between DataHow and Bristol Mayers Squibb.
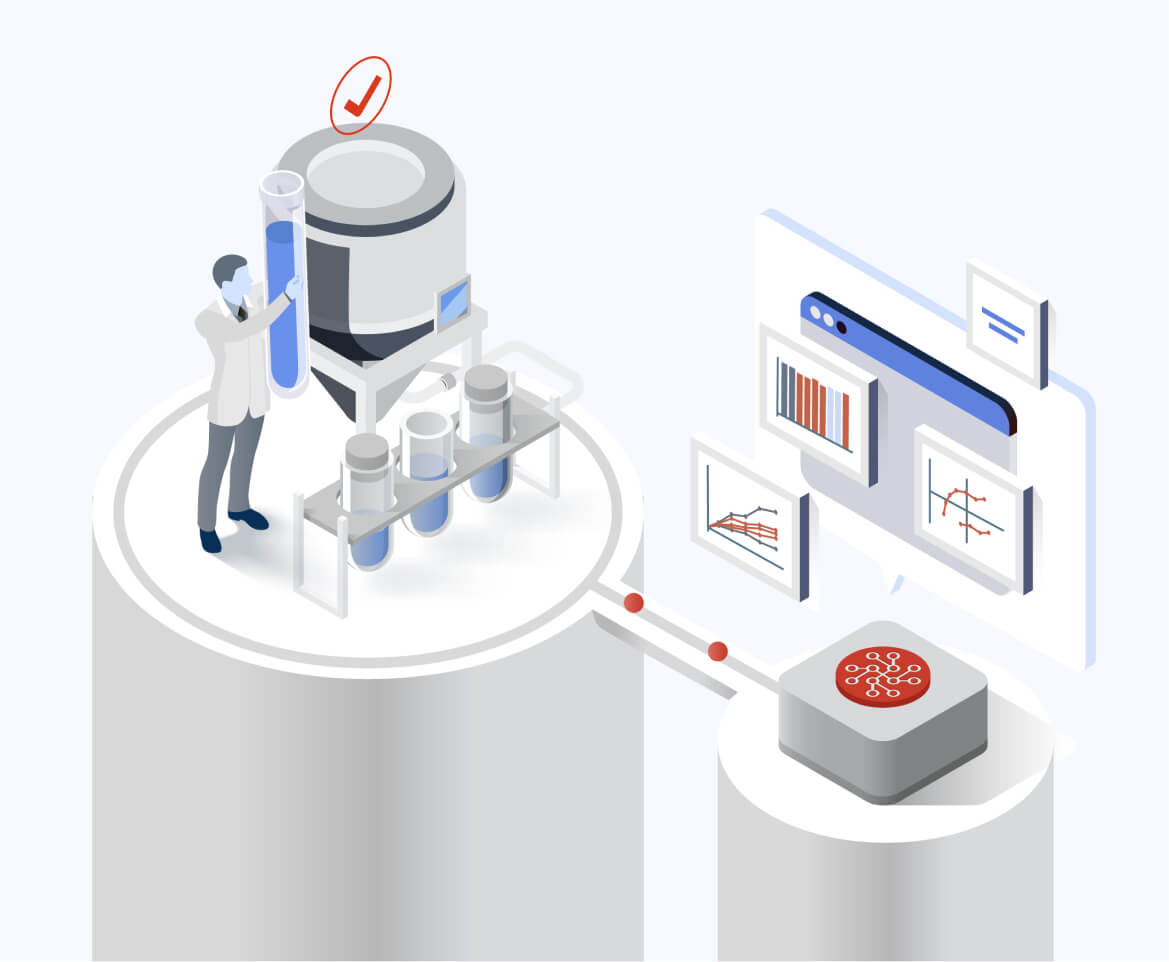
The Challenge and Technology Deployed
BMS designed and executed 48 (5-liter scale) experiments to evaluate the impact of 12 process parameters (CPPs) on 18 different product CQAs. To understand the complex interrelationships between the process parameters and CQAs, data from these experiments was analyzed using both industry “black box” multiple linear regression models and DataHows hybrid models.
DataHows hybrid models fuse mechanstic models, which algorythmically describe first principles knowledge for a particular process operation, and data driven models which learn purely from data. This powerful combination allows us to benefit from existing knowledge, while using machine learning to deliver insights where we lack understanding. Learn more about DataHow technologies.
Key Goals
- Evaluate the Ability of Hybrid Models to Predict CQAs
Compare hybrid models to industry black-box models in understanding and predicting the relationship between process parameters and Critical Quality Attributes (CQAs).
- Assess Experimental Data Requirements
Determine the number of experiments needed for each modeling approach to accurately predict CQAs (explained variance >60%).
- Assess Additional Benefits of Hybrid Models
Evaluate whether hybrid models offer any additional advantages over black-box models.
Key Results
The application of DataHowLabs hybrid modeling technology yielded immediate results and operational and process impact.
- An average 22% increase in predication accuracy accross the 18 CQAs
- 3x fewer experiments were required to accurately predict the 18 CQAs
- Hybrid models were able to deliver substantially richer process knowledge
To further explore the impact of the technology and a deep dive on the results, please download the full case study below. Should you wish to further explore the case with the DataHow team, please contact us directly.
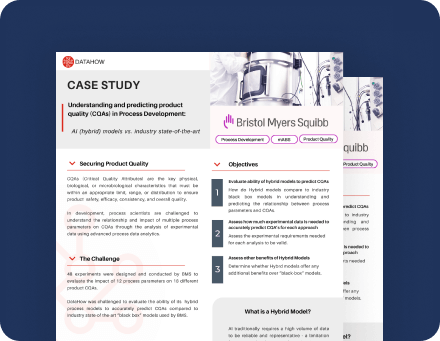